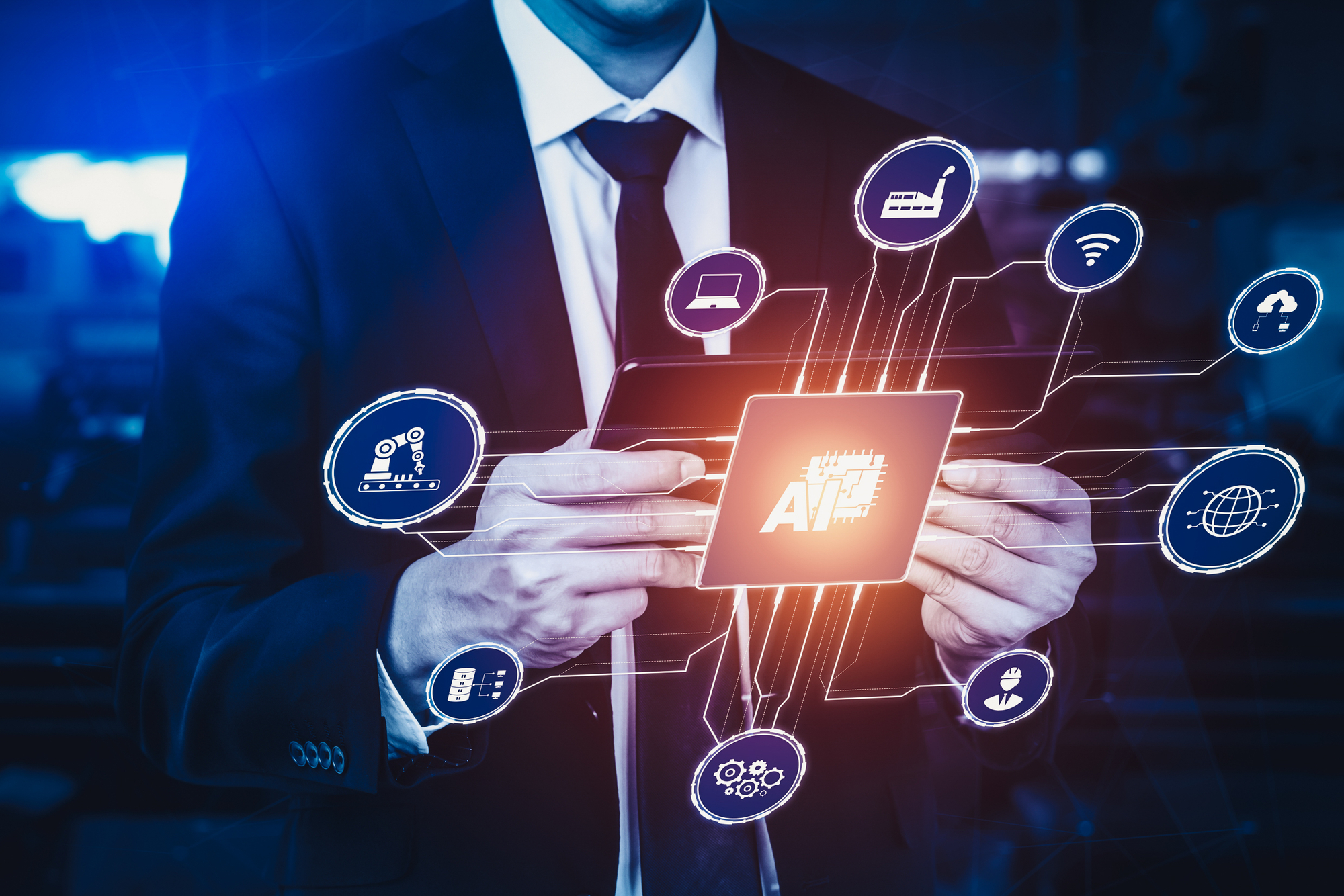
Artificial Intelligence (AI) is increasingly becoming a topic for discussions in the boardrooms of companies across the globe, and the manufacturing sector is no exception. Amid the myriad conversations about AI and machine learning, one particular aspect stands out clear to me: the undeniable link between accessing the right data and the ability to deliver AI capabilities. This connection is pivotal, but unfortunately missed very often. Where is the “data” is one of my questions and thoughts when I speak to developers of solutions and customers about AI. In other words, you need to put the data in before you get something out of these magic AI box to enhance decision-making processes in engineering and optimizing manufacturing operations.
CIMdata Webinar Insights
Earlier this month, I had the opportunity to attend a webinar hosted by CIMdata, titled “AI & PLM: Beyond all the Hype.” The webinar, led by Peter Bilello, the President of CIMdata, delved into the capabilities and landscape of AI solutions within the Product Lifecycle Management (PLM) domain. He discussed various sources and technologies of AI, machine learning, training data, deep learning, neural networks and their application to product lifecycle, manufacturing supply chain management, connecting to engineering and manufacturing processes.
I was looking for the answers how machine learning systems and machine learning technology can be applied to artificial intelligence systems to increase human intelligence in everything that is related to engineering, design, manufacturing planning, production, maintenance and support. In recent years, we’ve seen a big process in different applications of intelligence such as computer vision, machine learning models, generative AI systems. We are looking how computer science and AI will open new opportunity to make human brain more efficient – not to replace humans by to use input data and natural language processing to create capabilities to turn structured and unstructured data from engineering and manufacturing into machine learning model and neural network to support predictive analytics, decision support and other applications of AI.
My best part of the session was the discussion on identifying sources of intelligence in PLM-related data. From my perspective, pinpointing these sources is crucial. Peter Bilello highlighted four key areas: IoT-enabled data, the intersection of PLM and Enterprise Resource Planning (ERP), Manufacturing Execution Systems (MES), federated analytics and information lifecycle.
IoT Loops
The first source of intelligence comes from IoT (Internet of Things) loops. Data captured from various sources, particularly from products in use by customers, offers a rich source of information. This data is not only voluminous but also varied, require substantial computing power and sophisticated data processing capabilities to unlock its potential.
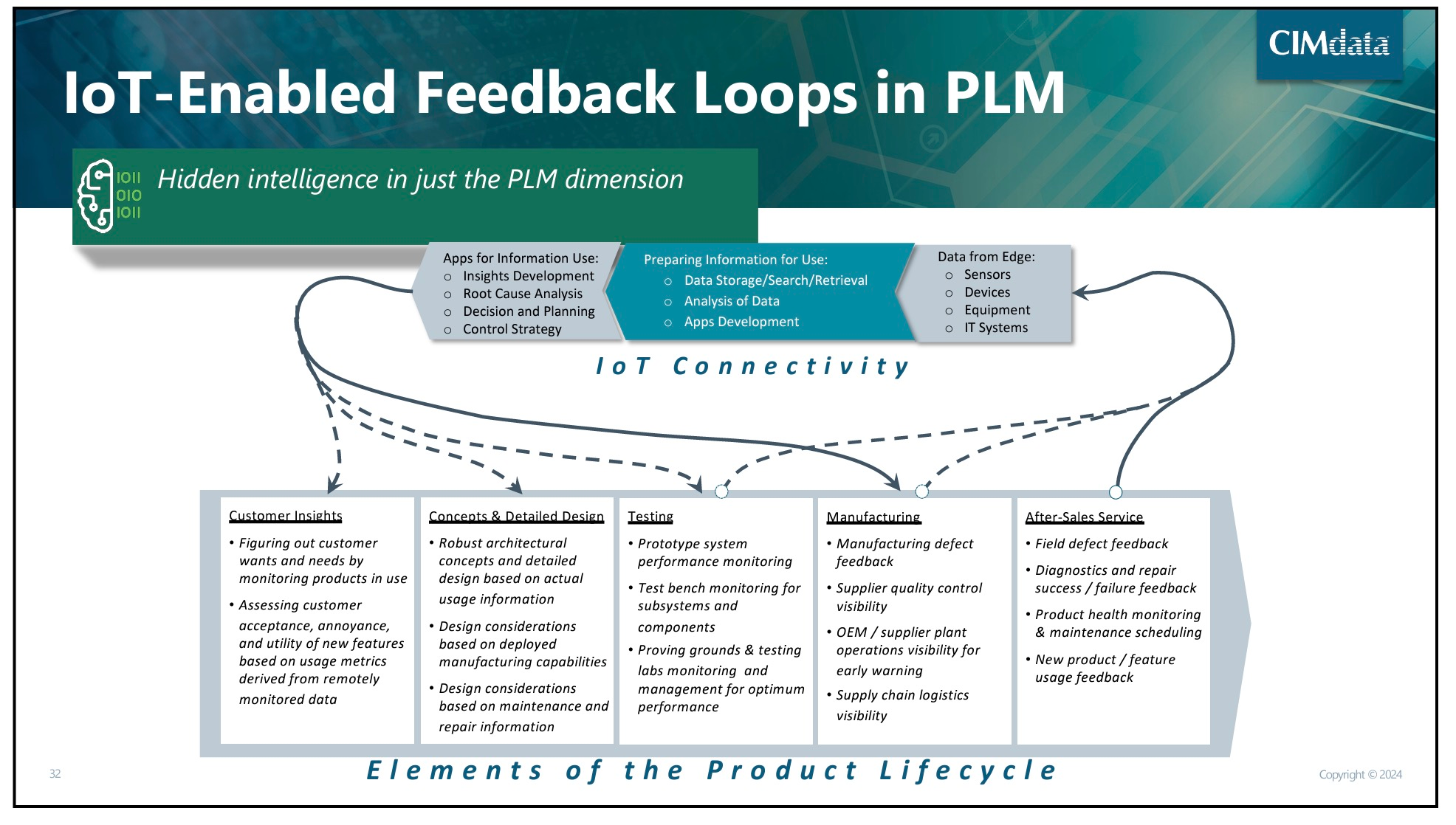
PLM/ERP Intersection
The convergence of PLM (intellectual assets) and ERP (business assets) is grabbing a significant attention. All companies I talk to are increasingly interested in connection between these two domains. The intersection becomes increasingly relevant. This need is driven by the growing number of connected manufacturing processes in the space of CTO and CTO+ (Configure To Order) processes.
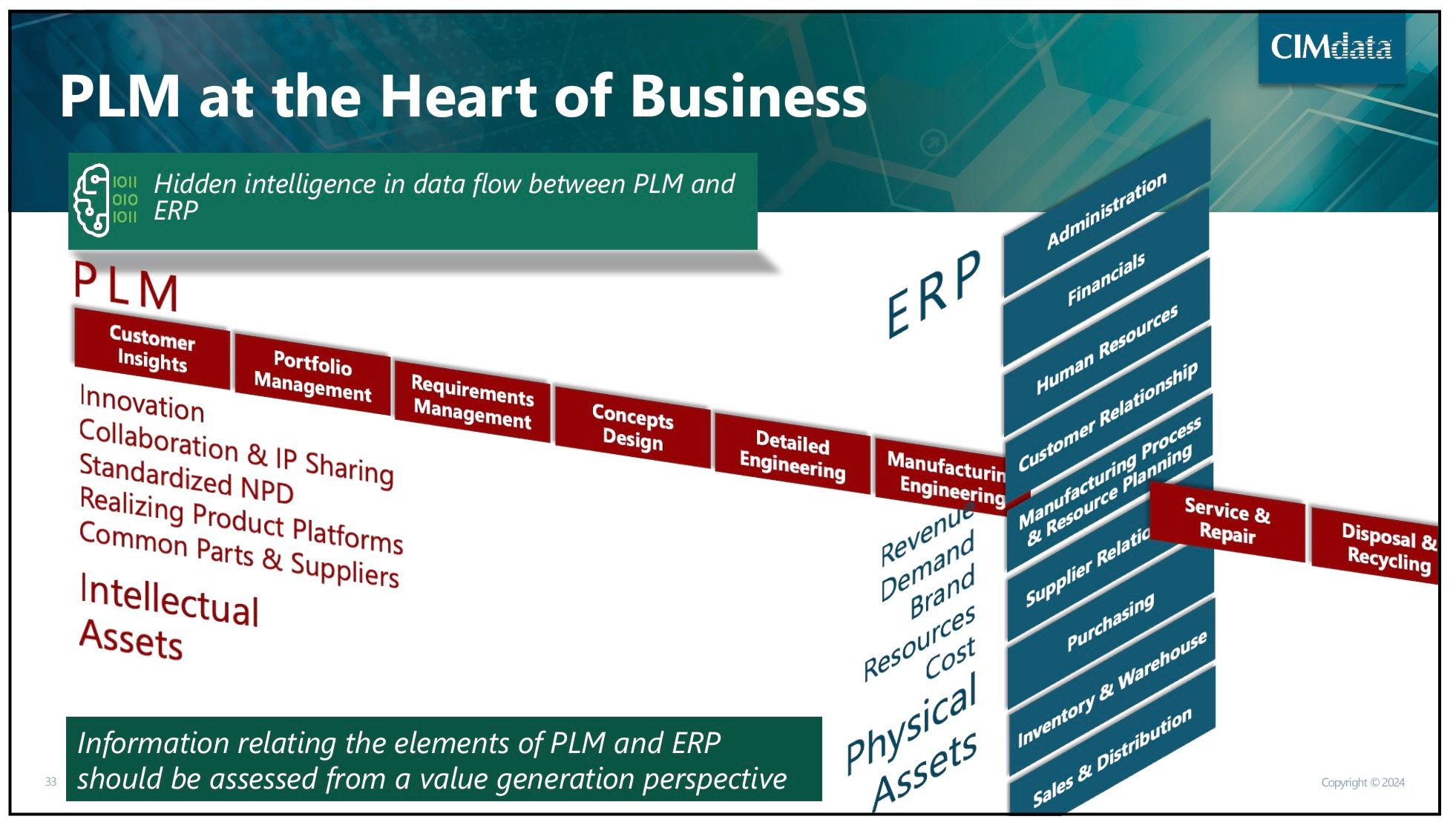
MES and Production Control
Another critical source of intelligence is MES and the automation and optimization of production planning. This includes a wide array of data – production test results, performance outcomes, material usage, product tracking and tracing, and personnel performance. The integration of this data with other systems like PLM, ERP, CRM (Customer Relationship Management), and HR (Human Resources) is another source of very interesting data.
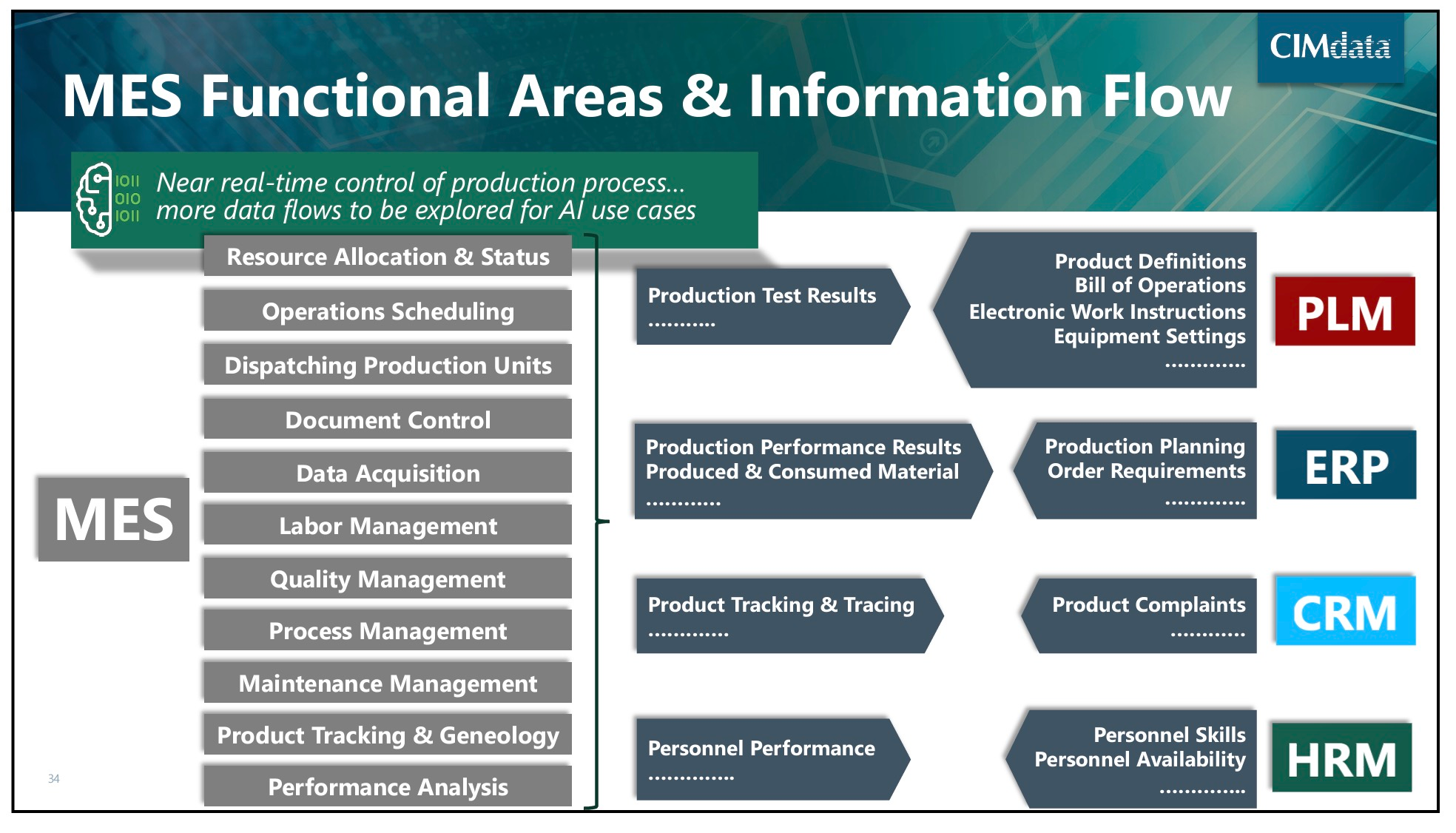
Analytic Data from Multiple Sources
The aggregation and analysis of data from disparate sources present a big opportunity to glean insights. This federated approach to analytics allows for a more nuanced understanding of operations and can inform more strategic decision-making. Analytic was a big topic recently in many organization and the data can be very valuable and probably easier to get (most of these implementations are new and many solutions are using cloud -based platforms.
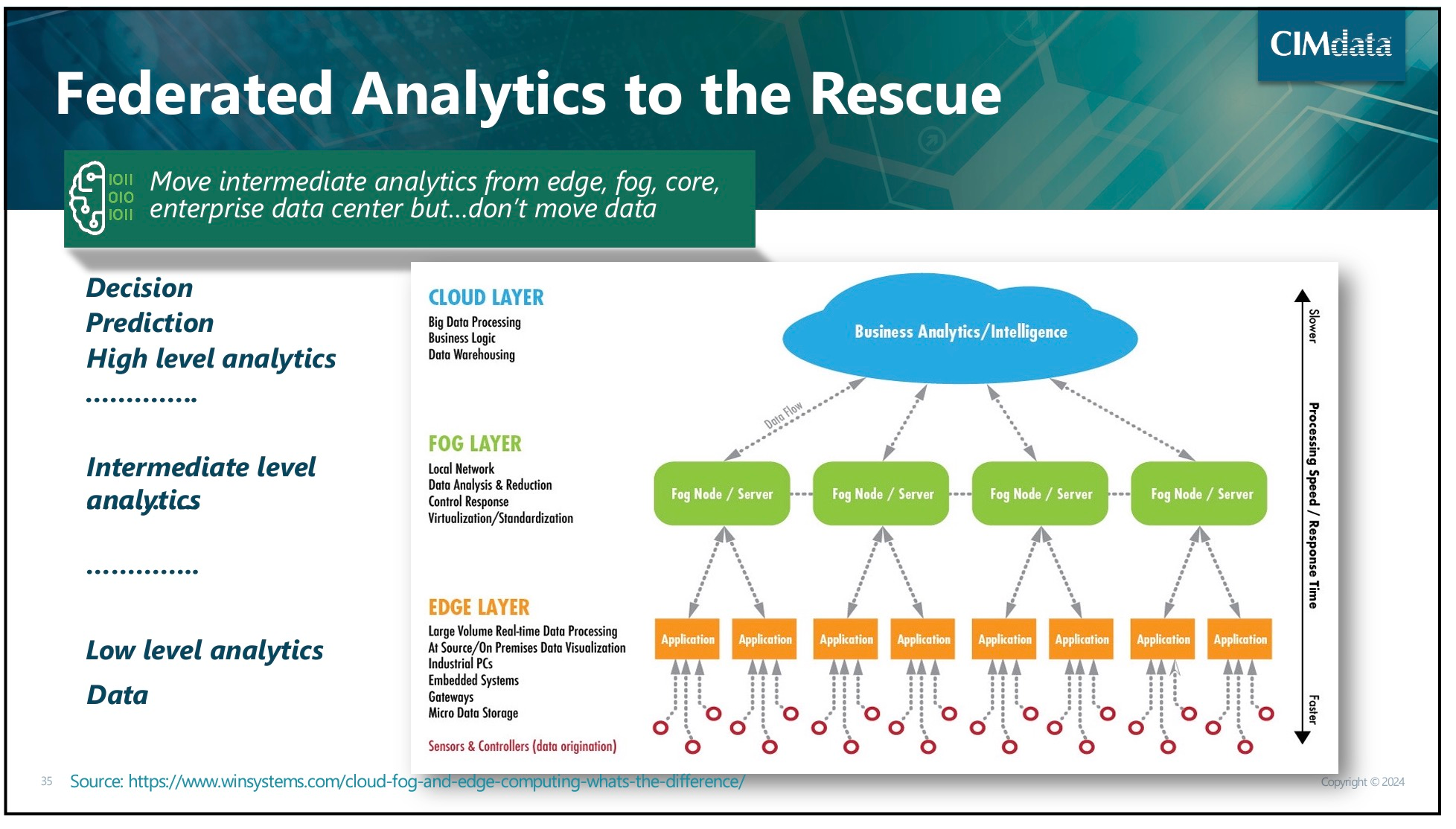
Information Flow Across Lifecycle Phases
The flow of information between various lifecycle phases offers a unique perspective on business processes and communication. It is easy and tough part at the same time. The domain of PLM was under increased pressure recently delivering digital threads – connectivity between various lifecycle phases and systems. The domain is a challenge by itself, but it can present and huge opportunity and data can be an important ground in the future PLM AI work.
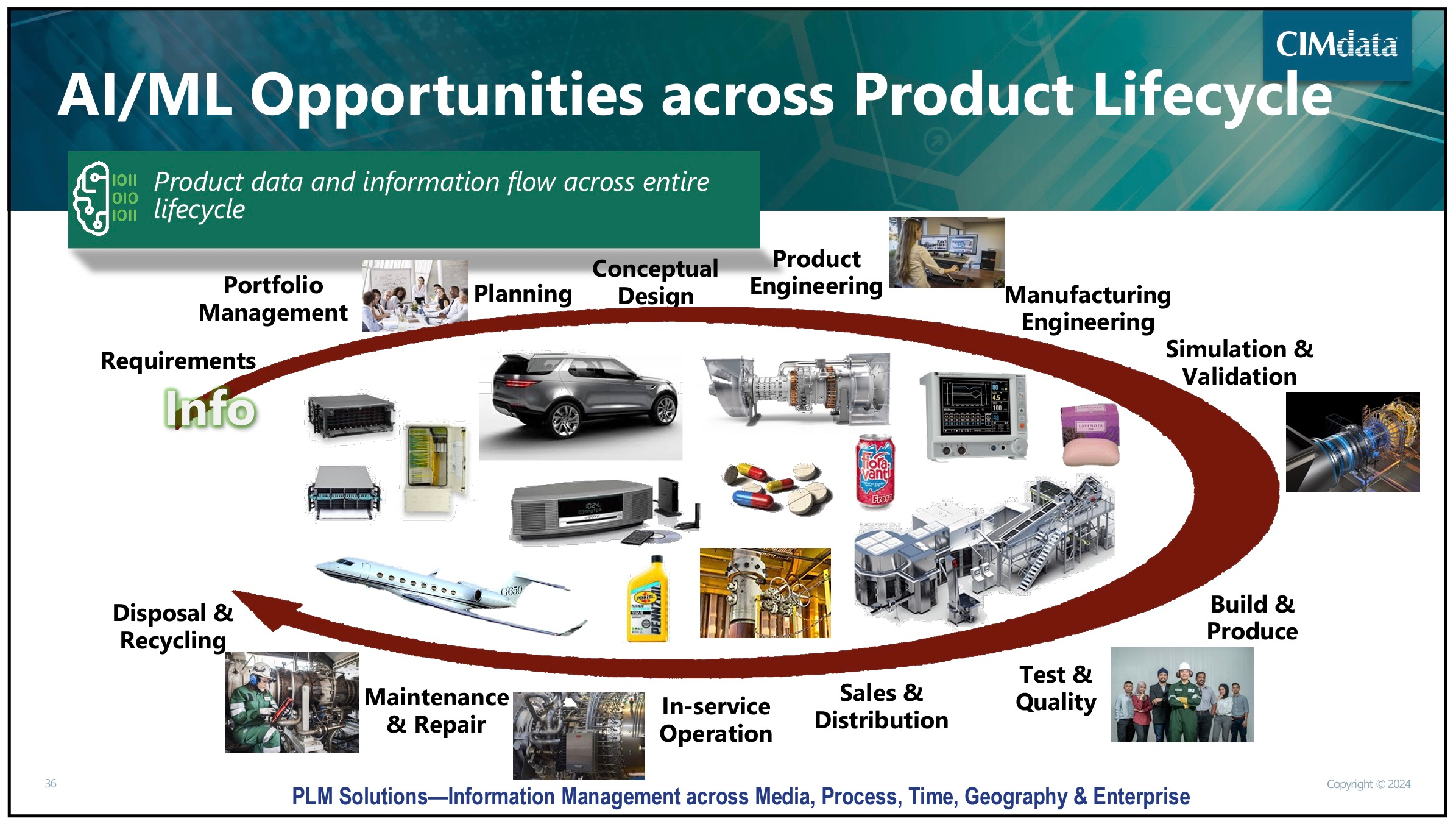
Are we going to see PLM AI systems any time soon?
The questions that everyone is asking these days- where and when are going to see AI systems in product lifecycle management. In my view, we are at the early days of AI exploration. Everyone is excited about what can happen. The last year ChatGPT and all followers expansions was super impressive. Every company I know today is developing some sort of “AI roadmap”.
I see the future of AI in product lifecycle management in a deep integrations of existing systems with some novel algorithms and capabilities focusing on efficiency (think about various ideas of co-pilots) and effectiveness of decision processes, including cost assessments, project progress, performance, risk mitigation and customer service improvements.
In my view, we are not going to see a “PLM AI system”, but we are going to see AI capabilities integrated in many places of existing system and processes. At the same time, I can see an opportunity for AI-enabled services that can be intertwined into existing enterprise systems to solve problems such as impact analysis, supply chain risk mitigation, cost assessment and many others.
Conclusion
The essence of AI in PLM lies in the data. The process of identifying the right sources of data, scoping this data accurately, applying it through AI technologies, and leveraging the results to bolster processes and decision-making is a complex yet rewarding journey. As I look to the future, the role of AI in PLM will only grow, powered by an ever-expanding pool of data and increasingly sophisticated analytical tools. The key to unlocking this potential lies in our ability to harness and interpret this data effectively, a challenge that, if met, promises to revolutionize the way we approach product lifecycle management.
To build an AI for product lifecycle management is multistep process cycling data exploration, analysis and practical experiments with customer data – this is an evolving landscape of PLM AI. Just my thoughts…
Best, Oleg
Disclaimer: I’m co-founder and CEO of OpenBOM developing a digital-thread platform with cloud-native PDM & PLM capabilities to manage product data lifecycle and connect manufacturers, construction companies, and their supply chain networks. My opinion can be unintentionally biased.
The post Sources of Intelligence for Future PLM AI- Thoughts after CIMdata webinar appeared first on Beyond PLM (Product Lifecycle Management) Blog.
Be the first to post a comment.